摘要: Digitization in the finance industry has enabled technology such as advanced analytics, machine learning, AI, big data, and the cloud to penetrate and transform how financial institutions are competing in the market. Large companies are embracing these technologies to execute digital transformation, meet consumer demand, and bolster profit and loss. While most companies are storing new and valuable data, they aren’t necessarily sure how to maximize its potential, because the data is unstructured or not captured within the firm.
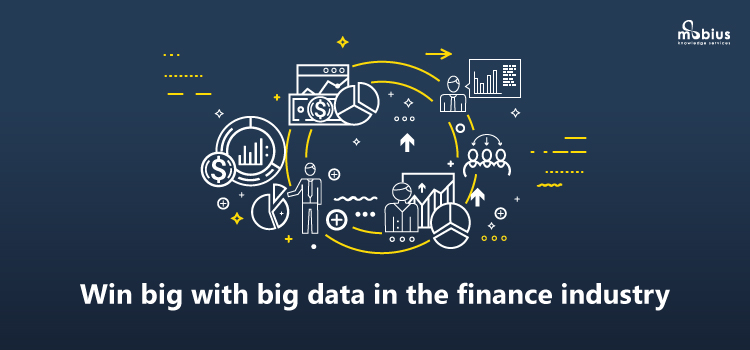
Our cutthroat digital age has started to implement AI applications in our everyday lives, various industries; and businesses, and sales are no exception. The way how AI is changing sales activities and strategies, affecting customer relations and developing partnerships between sales representatives and AI, recently became a fundamental buzz in the industry.Research shows that using intelligent technologies in the sales reporting practice can improve forecasting, one of the daunting challenges of working in this industry. When thinking about the potential that artificial intelligence can offer to this industry, AI can surely become one of the main resources for developing a 21-century sales strategy. Here is an overview of how AI can improve sales processes, and provide exceptional results.
As the financial industry rapidly moves toward data-driven optimization, companies must respond to these changes in a deliberate and comprehensive manner. Efficient technology solutions that meet the advanced analytical demands of digital transformation will enable financial organizations to fully leverage the capabilities of unstructured and high volume data, discover competitive advantages, and drive new market opportunities.
But first, organizations must understand the value of big data technology solutions and what they mean for both their customers and their business processes.
Big data in finance refers to the petabytes of structured and unstructured data that can be used to anticipate customer behaviors and create strategies for banks and financial institutions.
The finance industry generates lots of data. Structured data is information managed within an organization in order to provide key decision-making insights. Unstructured data exists in multiple sources in increasing volumes and offers significant analytical opportunities.
There are billions of dollars moving across global markets daily, and analysts are responsible for monitoring this data with precision, security, and speed to establish predictions, uncover patterns, and create predictive strategies. The value of this data is heavily reliant on how it is gathered, processed, stored, and interpreted. Because legacy systems cannot support unstructured and siloed data without complex and significant IT involvement, analysts are increasingly adopting cloud data solutions.
Cloud-based big data solutions not only cut costs of on-premise hardware with limited shelf life but also improve scalability and flexibility, integrate security across all business applications, and — most importantly — garner a more efficient approach to big data and analytics.
With the ability to analyze diverse sets of data, financial companies can make informed decisions on uses like improved customer service, fraud prevention, better customer targeting, top channel performance, and risk exposure assessment.
How big data has revolutionized finance.
Financial institutions are not native to the digital landscape and have had to undergo a long process of conversion that has required behavioral and technological change. In the past few years, big data in finance has led to significant technological innovations that have enabled convenient, personalized, and secure solutions for the industry. As a result, big data analytics has managed to transform not only individual business processes but also the entire financial services sector.
1.Real time stock market insights:
Machine learning is changing trade and investments. Instead of simply analyzing stock prices, big data can now take into account political and social trends that may affect the stock market. Machine learning monitors trends in real-time, allowing analysts to compile and evaluate the appropriate data and make smart decisions.
2.Fraud detection and prevention:
Machine learning, fueled by big data, is greatly responsible for fraud detection and prevention. The security risks once posed by credit cards have been mitigated with analytics that interpret buying patterns. Now, when secure and valuable credit card information is stolen, banks can instantly freeze the card and transaction, and notify the customer of security threats.
3.Accurate risk analysis:
Big financial decisions like investments and loans now rely on unbiased machine learning. Calculated decisions based on predictive analytics take into account everything from the economy, customer segmentation, and business capital to identify potential risks like bad investments or payers.
Applications of big data In finance.
Financial firms now have the ability to leverage big data for use cases such as generating new revenue streams through data-driven offers, delivering personalized recommendations to customers, creating more efficiency to drive competitive advantages, and providing strengthened security and better services to customers. Many finance companies are already doing big data right and getting immediate results.
1.Increased revenue and customer satisfaction:
Companies like Slidetrade have been able to apply big data solutions to develop analytics platforms that predict clients’ payment behaviors. By gaining insight into the behaviors of their clients a company can shorten payment delay and generate more cash while improving customer satisfaction.
2.Speeding up manual processes:
Data integration solutions have the ability to scale up as business requirements change. Access to a complete picture of all transactions, every day, enables credit card companies like Qudos Bank to automate manual processes, save IT staff work hours, and offer insights into the daily transactions of customers.
3.Improved path to purchase:
Legacy tools no longer offer the solutions needed for large, disparate data and often have limited flexibility in the number of servers they can deploy.
Cloud-based data management tools have helped companies like MoneySuperMarket get data from several web services into data warehouses for consumption by various departments, such as finance, marketing, business intelligence, market intelligence, and reporting. Cloud strategies like these improve the path to purchase for customers, enable daily metrics and performance forecasts as well as ad hoc data analysis.
4.Streamlined workflow and reliable system processing:
Ever-rising data volumes in banking are leading to the modernizing of core banking data and application systems through uniform integration platforms. Matched with a streamlined workflow and a reliable system for processing, companies like Landesbank Berlin have applied application integration to process 2TB of data daily, implement 1,000 interfaces, and use just one process for all information logistics and interfacing.
5.Analyze financial performance and control growth:
With thousands of assignments per year and dozens of business units, analyzing financial performance and controlling growth between company employees can be complex. Data integration processes have enabled companies like Syndex to automate daily reporting, help IT departments gain productivity, and allow business users to access and analyze critical insights easily.
Four big data challenges in finance.
As big data is rapidly generated by an increasing number of unstructured and structured sources, legacy data systems become less and less capable of tackling the volume, velocity, and variety that the data depends on. Management becomes reliant on establishing appropriate processes, enabling powerful technologies, and being able to extract insights from the information.
The technology is already available to solve these challenges, however, companies need to understand how to manage big data, align their organization with new technology initiatives, and overcome general organizational resistance. The specific challenges of big data as related to finance are a bit more complex than other industries for many reasons.
1. Regulatory requirements.:
The finance industry is faced with stringent regulatory requirements like the Fundamental Review of the Trading Book (FRTB) that govern access to critical data and demand accelerated reporting. Innovative big data technology makes it possible for financial institutions to scale up risk management cost-effectively, while improved metrics and reporting help to transform data for analytic processing to deliver required insights.
2. Data security.:
With the rise of hackers and advanced, persistent threats, data governance measures are crucial to mitigate risks associated with the financial services industry. Big data management tools ensure that data is secure and protected, and that suspicious activity is detected immediately.
3. Data quality.:
Finance companies want to do more than just store their data, they want to use it. Because data is sourced from so many different systems, it doesn’t always agree and poses an obstacle to data governance. Data management solutions ensure information is accurate, usable, and secure.Simultaneously, real-time analytics tools provide access, accuracy, and speed of big data stores to help organizations derive quality insights and enable them to launch new products, service offerings, and capabilities.
4. Data silos.:
Financial data comes from many sources like employee documents, emails, enterprise applications, and more. Combining and reconciling big data requires data integration tools that simplify the process in terms of storage and access. Big data solutions and the cloud work together to tackle and resolve these pressing challenges in the industry. As more financial institutions adopt cloud solutions, they will become a stronger indication to the financial market that big data solutions are not just beneficial in IT use cases, but also business applications.
How to get started with big data In finance.
Large financial firms have paved the path towards big data adoption and provided proof that big data solutions are real. Each financial company is at their own level of big data application and maturity, but the core drive towards full adoption originates from the same question all across the board: “How can data solve our top business problems?” Whether the core issue is customer experience, operational optimization, or improved business processes, there are certain steps that financial organizations must take to fully embrace the data-driven transformation that big data and cloud-based solutions promise.
1. Define a data strategy.:
Defining a data strategy should always start with a business goal. A comprehensive strategy will span across all departments, as well as the network of partners. Companies must examine where their data is heading and growing, instead of focusing on short-term, temporary fixes.
2. Select the right platform.:
The needs of each business are different. Selecting a cloud data platform that is both flexible and scalable will allow organizations to collect as much data as necessary while processing it in real-time. More importantly, the finance sector needs to adopt a platform that specializes in security. Tracking data at a granular level and ensuring that valuable information is accessible to key players will make or break a data strategy.
3. Start with one problem.:
Big data has a lot of capabilities. Identifying and tackling one business challenge at a time and expanding from one solution to another makes the application of big data technology cohesive and realistic. Basic use cases can easily be built upon and expanded over time.
......
Full Text: talend
Related Articles:
The Vital Elements of a Big Data Branding Strategy
In Depth: China’s Big Data Clampdown Leaves Online Lenders in a Bind
Bombardier brings big data to business aviation
How big data and AI work together
當數位轉型成為一股無法抗拒的浪潮,組織該如何調整才能「站在浪頭上」!
若喜歡本文,請關注我們的臉書 Please Like our Facebook Page: Big Data In Finance
留下你的回應
以訪客張貼回應